Table Of Content
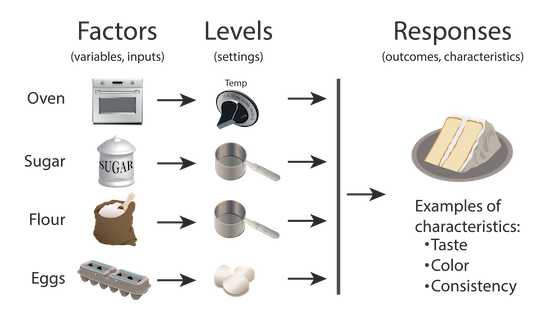
Regardless of who or what is involved in the process - it is still going to work. We will come back to this notion of robustness later in the course (Lesson 12). As an example of a one-factor experiment, data from an incoming shipment of a product is given in Table 1. Specify how you can manipulate the factor and hold all other conditions fixed, to insure that these extraneous conditions aren't influencing the response you plan to measure. The same problems of having “low resolution” will apply to your DOE design.
Blocking
Two of the most common approaches to DOE are a full factorial DOE and a fractional factorial DOE. Let’s start with a discussion of what a full factorial DOE is all about. In 1950, Gertrude Mary Cox and William Gemmell Cochran published the book Experimental Designs, which became the major reference work on the design of experiments for statisticians for years afterwards. You can also compare different levels for given factors, such as whether a cultivar from nursery A produces a higher yield, better taste, or both than a plant from nursery B. Strawberries also need plenty of water to ensure juiciness; applying 1ml of water would be difficult to accurately achieve and, possibly, trigger drought stress responses.
Optimization
It incorporates a lot of previous statistical and management techniques. Robustness is a concept that enters into statistics at several points. At the analysis, stage robustness refers to a technique that isn't overly influenced by bad data. Even if there is an outlier or bad data you still want to get the right answer.
By KnowledgeHut .
Around 1990 Six Sigma, a new way of representing CQI, became popular. Now it is a company and they employ a technique which has been adopted by many of the large manufacturing companies. This is a technique that uses statistics to make decisions based on quality and feedback loops.
One factor at a time (OFAT) method
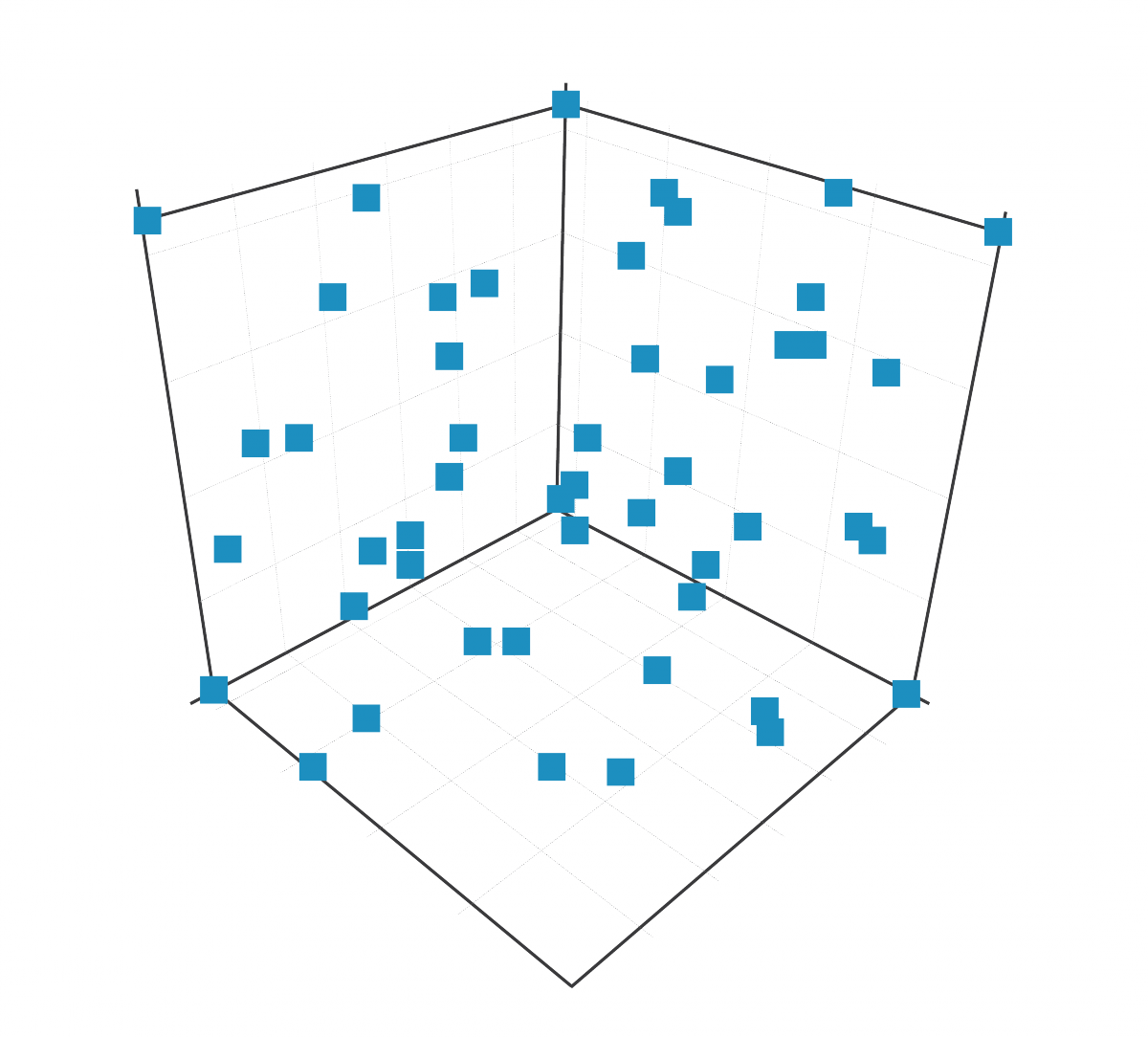
However, the nature of the independent variable does not always allow for manipulation. In those cases, researchers must be aware of not certifying about causal attribution when their design doesn't allow for it. The same goes for studies with correlational design (Adér & Mellenbergh, 2008).
This is when you might choose to run a fractional factorial, also referred to as a screening DOE, which uses only a fraction of the total runs. That fraction can be one-half, one-quarter, one-eighth, and so forth depending on the number of factors or variables. Design of Experiments is a framework that allows us to investigate the impact of multiple different factors—changed simultaneously—on an experimental process. A perfect cup of tea depends on multiple other factors, such as the blend, brewing time, and the addition of sugar. In other words, making a perfect cup of tea is complex and multidimensional.
Components of Experimental Design
In this second experimental series, the pH is changed from 2.5 to 5.0 and you can see the measured yields. In order to understand why Design of Experiments is so valuable, it may be helpful to take a look at what DOE helps you achieve. A good way to illustrate this is by looking at an alternative approach, one that we call the “COST” approach. Another important application area for DOE is in making production more effective by identifying factors that can reduce material and energy consumption or minimize costs and waiting time. It is also valuable for robustness testing to ensure quality before releasing a product or system to the market.
Design of experiments as a tool to guide the preparation of tailor-made activated carbons Scientific Reports - Nature.com
Design of experiments as a tool to guide the preparation of tailor-made activated carbons Scientific Reports.
Posted: Thu, 09 Mar 2023 08:00:00 GMT [source]
As well as these savings, DOE achieves higher precision and reduced variability when estimating the effects of each factor or interaction than using OFAT. It also systematically estimates the interaction between factors, which is not possible with OFAT experiments. In a series of blogs, we’re going to explore the basis of DOE, who should consider DOE, and some ways in which this methodology helps experimental biologists deal with life’s inherent complexity.
Process Improvement
Our school teachers advocated a one-factor-at-a-time (OFAT) approach to scientific experimentation. So, pick a variable (factor) and vary the value (levels), while keeping everything else constant. Each of these factors will have an impact on the final outcome of interior decoration. While variation in each factor alone can impact, a variation in a combination of these factors at the same time also will impact the final outcome. Once completed, Design of Experiments helps the Six Sigma project team better identify the combination of inputs that lead to the highest-quality product or service.
It also helps remove complexities and streamlining the design process for cost management in the manufacturing process. Full factorial designs investigate all possible combinations of factors and levels. Full factorial designs, however, involve a large number of runs, increasing exponentially as the number of factors increase. The Design of Experiments (DoE) landscape is rich with diverse strategies tailored to uncover specific insights within various research domains. At the foundation, we have basic designs such as the completely randomized design and the randomized block design, which serve as the starting points for most experimental frameworks.
Compared to other experimental approaches, DOE saves time and resources when performing experiments, whilst providing deeper insight into complex systems. The method was coined by Sir Ronald A. Fisher in the 1920s and 1930s. Design of Experiment is a powerful data collection and analysis tool that can be used in a variety of experimental situations. Optimal performance of your process is locked inside current performance, just waiting to be discovered. The optimal process can emerge once all the variables are adjusted appropriately.
At any stage in your DOE campaign, you could take your pick from several designs, depending on your assumptions, goals, available run numbers, and so on. The beauty of DOE is that by choosing a DOE design, you have also chosen the type of analysis you will do. By picking the right design in the beginning, you’ll be saving yourself tons of work and grief when it comes to the analysis. Which means that you don’t have to design a single experiment—or pick a single DOE design—that will answer all your questions. So, for example, first we might fix the pH at 3, and change the volume of the reaction container from a low setting of 500ml to a high of 700ml. Additional content around the benefits of subscribing to this blog feed.
No comments:
Post a Comment